Complex-Valued Deep Neural Networks for Brain Tumor Characterization in MRI k-Space data
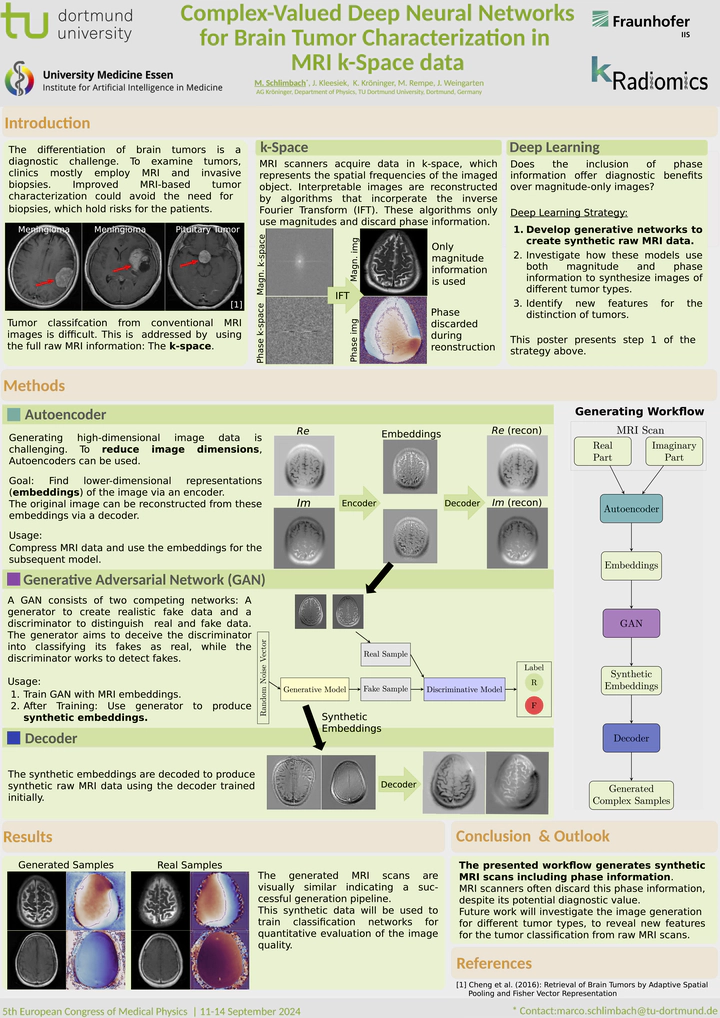
Introduction: Differentiating between brain tumors is diagnostically challenging, but crucial for treatment decisions. Their identification includes methods like biopsies and Magnetic Resonance Imaging (MRI). Previous studies employed various Machine Learning techniques to enhance MRI-based tumor classification, aiming to avoid invasive biopsies. These methods typically use the final MRI images, which are the result of a complex reconstruction pipeline involving filtering operations. This reconstruction discards the phase information from the raw MRI data, known as k-space data, which might hold significant diagnostic value. Our project applies classifiers and generative methods directly in the complex valued k-space to identify new features for tumor analysis.
Materials & Methods: A range of Complex Valued Deep Neural Networks (CVDNNs) is employed, including Generative Adversarial Networks and autoencoders for synthetic data generation, alongside convolutional networks and U-Nets for preprocessing and classification. The FastMRI dataset [1] from NYU Langone and Facebook AI Research is used for training.
Results: Initial studies show the feasibility of applying Deep Learning to k-space. A complex valued U-Net successfully performs skull stripping for brain MRI scans [2]. In the generative approach, investigations show that it is potentially possible to produce data directly in the k-space domain.
Summary: The project innovates medical imaging and brain tumor diagnosis, transitioning from standard processed images to applying Deep Learning on raw MRI data. The use of CVDNNs in processing this raw data marks a significant change in how MRI scans are analyzed.